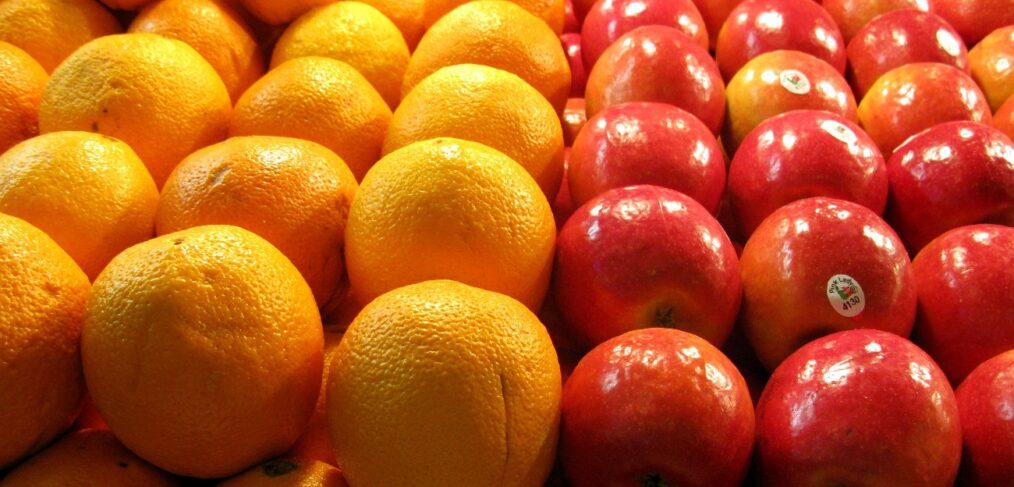
THE ART OF THE MERGER: a Music Metadata QC Crash Course
This month we take a quick tour through the world of Metadata Quality Control, the most exciting way to enjoy a life lived in spreadsheets. We focus on problems that arise as libraries grow larger and inconsistencies abound, with our signature TTA tips and tricks to help you make sense of the mess.
Working with data is fun, in the same way cleaning out your garage can be fun.
And if you’re like me and don’t even want to look at your garage right now, then you also know what a nightmare it can become.
Just as weekend projects and good intentions inevitably run their course and wind up fire hazards, music metadata all too often meets the same messy end – as libraries combine, new artists are signed, opportunities emerge and midnight oil burns. In no time, the pristine sanctitude of your musical walled garden transforms before your eyes into an unsightly tangle of weeds, gopher holes, and worst of all: fruit dying on the vine.
So what do you do?
Maybe it’s telling that I started this article with the phrase “Working with data is fun,” but hear me out. The sincere joy of turning a hopeless mess into a gleaming inspiration is not something I expected to list as one of my favorite things to do, but here we are.
Welcome to Metadata QC.
PART 1: Human Error
Being overwhelmed by data is the most human response you can have when trying to ingest content that is formatted specifically for computers to read. It can be helpful to remember that in the case of descriptive metadata, all entries in a spreadsheet (and therefore all potential sources of error) do in fact come from people, and likewise can be understood as a collection of people’s actions.
Take for example a common problem in quickly growing music libraries:
Poorly Assigned Tags
You try out some search terms on SourceAudio (for fun, or at the behest of an underwhelmed subpublisher), and suddenly you’re flooded in a sea of irrelevant music, track after track flowing over with a cornucopia of inane metadata tags.
After working through the initial urge to hit delete on the metadata and start over from scratch, I would suggest that your second response might be to look for the *human* pattern:
- Are any of the problematic tracks from the same album? Same playlist/batch/upload timeframe?
- If so, who tagged that album/etc.?
- Did they tag any other albums? Are the other albums as bad?
- (If so, is it time to find a new tagger?)
- If not, is it something specific you can address?
This isn’t meant to be pedantic – the point here is to turn data problems into human problems, which, unsurprisingly, are easier for humans to solve.
It doesn’t take a degree in data science to start picking apart where things might be going wrong with your data, and in fact, staring at data before you even know what you’re looking for could actually impede your understanding of what’s really going on. Arming yourself with even the most basic conjecture of why your metadata might be the way it is gives you a path through the data, a path that may very well give you a better sense of the scope of your problems.
Again, for those who missed it:
Staring at data before you even know what you’re looking for could actually impede your understanding of what’s really going on.
Take another symptom of the same issue: tracks with reasonable numbers of tags, but with tags that feel “off”.
Start again with a simple diagnosis:
- Is there a list of specific tags that seem to be used inaccurately/appearing too frequently?
- Again, is there a pattern to where these tags show up indicating a specific person misusing a tag?
– OR – - If there’s no info on who tagged your music, can you quarantine a specific pattern of usage to a set of albums/playlists etc.?
Even in this simple case, breaking down a categorical problem (bad/misused/poorly assigned tags in the library) into a specific problem (albums x- through y- have a problem with tags a, b, c) simplifies the task of fixing it down to a much more manageable level, opening the door to perhaps a small selective retag, or a few (very careful) find-and-replace operations on a subset of the data.
PART 2: Systemic Problems
Of course, metadata QC issues are rarely as simple as we’d like them to be.
And when the structure of libraries change quickly, as they often do via merger with another library, addition of large new catalogs, or integration with new distributors, the integrity of existing data can very easily be compromised by seismic shifts in the fundamentals of your music search.
SPLINTERING and MULTIPLE TAXONOMIES
Or, the “Apples and Oranges” problem.
Alternate usage of search terms (think of the differences between the words “Happy”, “Joyful”, and “Elated”, or “Sad”, “Depressed”, “Melancholy”) are exceedingly common in libraries with more than one person writing descriptive metadata. When assigned consistently, these nuances add depth to search that can provide real correlation between tracks spanning albums, catalogs, and musical styles, and ultimately offering a rich discovery experience for those doing the searching.
Apply inconsistently though, and you get the exact opposite: entire albums, artists, or catalogs siloed into their own independent search realms, with few tags from one corner of your library interrelating to any of the others, and ultimately offering little invitation for music editors or other listeners to explore the depth of your repertoire. Otherwise diverse music libraries suffering from splintered taxonomies can wind up with bland, samey-sounding search results that obscure the true breadth of the music within.
So, what do you do?
Without taggers to blame (and assuming the tracks were tagged consistently), instead we have to turn to the way the taxonomy is applied:
- Are you noticing different tags across different tracks that could be consolidated?
(Think outdated terms, the redundant usage described above, synonyms or overly-nuanced terms) - Starting small, can you identify clusters of tags that mean the same thing, but are used differently across different tracks or catalogs?
- Are you noticing systemic differences between the way terms are applied?
(Think subpublishers of years past with their own specific search terms, applied on specific parts of your library) - Are there catalog-level changes you could apply to your taxonomy as administered to improve the way tracks of different sources and origins relate to one another?An important note: it’s fine to want a diverse spread of search terms on individual tracks (to account for the different words people may use to search for specific moods or feelings); the point here is to have tags applied consistently, something all but impossible if none of the words are used the same way. If working with a platform that doesn’t automatically diversify search terms, add these extra words back in after you know they’ll be applied evenly across the board.
It goes without saying that operations to perform these changes should be executed with the utmost care, and with consideration for the types of nuance that are inevitably lost in a consolidation effort. There exists a spectrum between total uniqueness and total sameness where tracks in a library can either drown in uniformity or wither in obscurity. Finding the sweet spot where your music naturally assembles itself into trends, correlating deeply with the works surrounding it, is where the true beauty of optimized music metadata begins.
Tagteam Analysis offers music tagging and metadata services to companies and libraries in need of advanced music search tools and optimization. Read more about us on our website: tagteamanalysis.com