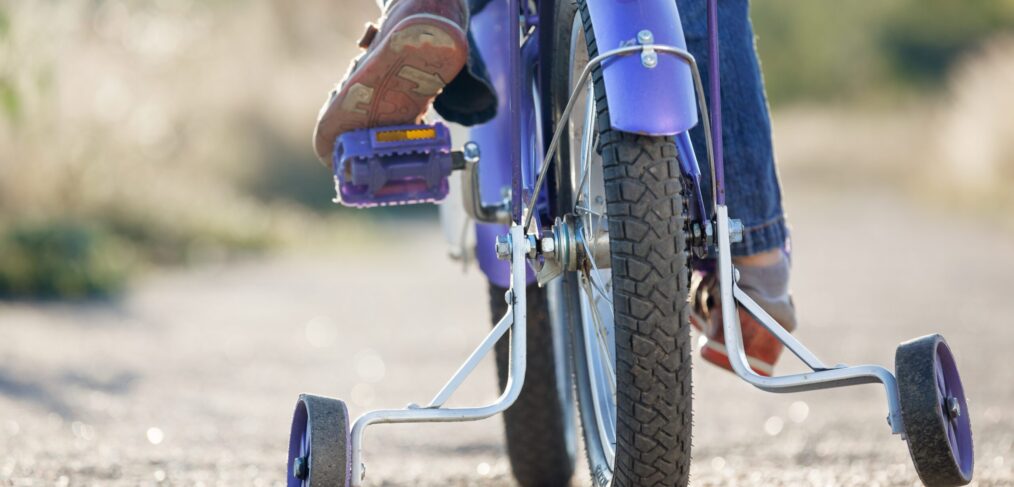
AI In Training: Data In, Data Out
This month, we talk about the importance of quality metadata in the highly anticipated and hotly debated field of Machine Learning and Artificial Intelligence. For more information on our services as the top human-tagged music metadata company in the production music industry, see our about page.
The music industry has every reason to be skeptical of AI.
Between the largely empty promises of crypto, Web3, the metaverse, or any number of other highly hyped and bewilderingly valued Big Tech initiatives over the last few years, the average person could hardly be blamed for a healthy level of fatigue (if not outright disdain) for the AI Arms Race we find ourselves in now.
Add to that the historic Hollywood Writers’ Strike, which positioned an entire industry firmly against the burgeoning threat of ChatGPT, or the near complete collapse of NFTs as anything resembling a vehicle for artistic empowerment. All this against a backdrop of AI-powered products and AI-generated content now flooding the internet (with the ensuing copyright ramifications), and it’s hard not to feel like AI has a lot to prove to creatives right now.
We at TTA have tried to reserve a measured interest as AI technologies have slowly found their way into the production music industry. But with big recent AI announcements from Google, OpenAI, and Nvidia (with presumably Apple to come at WWDC in June), we feel it’s time we take a moment to reflect on the technology, its promises, and how we think it could be made better despite its many limitations.
So what makes AI good? And what does it take to train AI that is great? Let’s dive in.
Part 1: Training Wheels
For the last year, generative AI has taken the internet by storm, improving in capability and utility at what feels like an unstoppable, breakneck pace.
But as exciting and powerful as AI products like ChatGPT, Google Gemini, DALL•E, Midjourney, Sora, or Microsoft Copilot are, it’s worth noting that they all share a common core technology dependent entirely on vast amounts of training data.
Large Language Models, as their name implies, must be trained on a large corpus of text data, with top performers in this category like OpenAI’s GPT-4o and Meta’s Llama 3 opting to train on vast swaths of copy scraped directly from the internet (like this article). The same goes for the models powering generative AIs that produce images, video, or even music, with companies like Udio or Suno being the latest to turn out strikingly musical results based on simple text prompts and lyrics.
The matter of where all this image, video, or music data is sourced from continues to escalate into a serious problem for the AI industry. But it remains the case that training any text-based cross-modal AI model (i.e., text-to-image, text-to-speech, or text-to-music) requires, by necessity, large amounts of accurate descriptive metadata. Through simulated human lifetimes of machine learning, often accelerated by the world’s fastest GPUs, AI Models in training compare image, video, audio, or any other data against its annotated metadata to build an embedded space—a numerical understanding of how to predict what word or sequence of words corresponds with any potential selfie, drawing, cat video, or blip of sound.
Crucially, the accuracy of this training metadata is absolutely paramount, as it forms the basis for the AI’s entire understanding of the world. Just like if you told the smartest person in the world that the color Red was actually Green, or that Sour was Sweet, an AI model trained on inaccurate (or biased/skewed) data will reflect those misunderstandings, happily parroting them back without a second thought.
As a result, the AI industry is now finding itself in a datamining gold rush, with tech giants scrambling to secure access to the best (and most accurate) training data while more and more companies, artists, creatives, and rights owners opt to restrict access to their personal wealth of human-generated material.
Part 2: Garbage In, Garbage Out
This last part is where things start to get sticky for the hype narrative around AI.
Because, for as powerful and provocative as many of these AI models are, much of the excitement for them is based on the promise of the technology—both in terms of its expected accuracy and its real-world usefulness.
Despite known issues like hallucination in LLMs (i.e., imagining facts that don’t exist), bizarre and unnerving visual artifacts in image and video-generation AIs, and the often robotic or lackluster (though nonetheless fascinating) results in music generation, the promise is that with enough data, all these things will only necessarily improve. Some even speculate that with enough data, we will reach a machine-intelligence explosion precipitating the creation of AGI—a mythical hyperintelligent AI that is powerful enough to help humanity in ways scarcely imaginable to us today.
All of these potential outcomes, however, bank on there being lots and lots of novel, relevant, and highly accurate metadata on whatever subject we need a potential AI to understand. How much data it will take to get there, though, is still entirely speculation, with some researchers pointing to studies that suggest generative AI has already peaked. Meanwhile, this growing demand for quality, human-generated training data is made even more complicated as AIs in the wild today continue to pump out low-quality content at a speed that clutters potential datasets with derivative AI works and could easily outpace the work of authentic human creators.
Part 3: Quality In, Quality Out
Those who know TTA may now see why this topic is close to our heart.
For the last ten years, our core mission as a human-tagged music metadata company has always been about making sure technology works for humans, not the other way around. Any skepticism we bring to the table comes mostly from a place of wanting to make sure the technology is actually useful—not more trouble than it’s worth.
With every indication that AI is becoming the new search, it only makes sense that our expertise in search tagging for production music would remain relevant. And with the increasing necessity for human input in the AI training loop, we see our roster of highly trained analysts and proprietary tagging methods that allow us to provide quality music metadata at scale as more valuable than ever. We’ve also seen growth in our business over the last few years working with large stock music and image catalogs (Shutterstock and Epidemic Sound, to name two) in need of this exact kind of high-volume, high-accuracy music metadata for ML training purposes.
Having cut our teeth tagging music metadata for many major production music catalogs, we take pride in our expertise at identifying Genre, Mood, Texture, Instrument, Lyrical Theme and Industry Usage tags, even as our AI competitors (some of which we helped train) struggle to reach the level of accuracy required for high-performance music libraries. And given the ever-changing nature of musical styles in culture, we only anticipate our expert analysis becoming increasingly important to music libraries and AI companies alike in the future.
For more information on the metadata services we provide, feel free to contact us to discuss your project.